Flavour
Opinion: With so many ‘flavours’ of AI, food and beverage brands must choose wisely
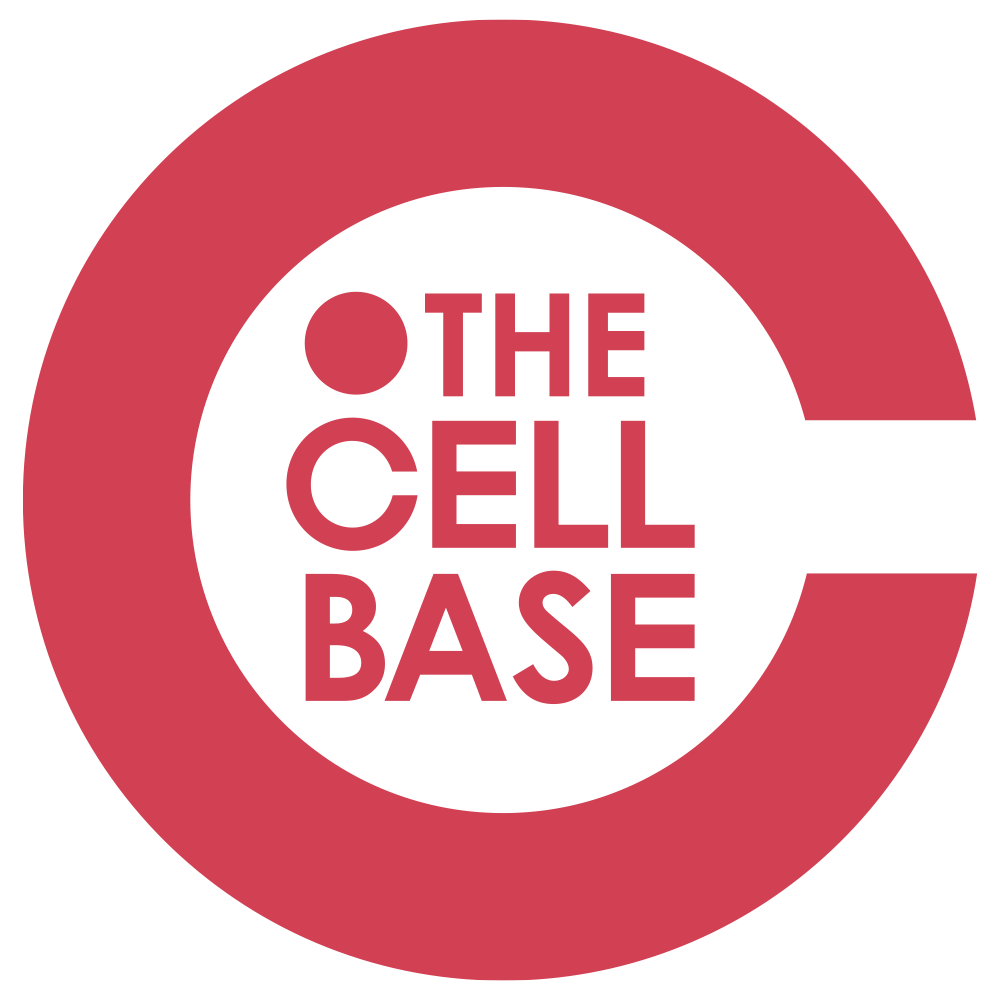
Guest
20 April 2025
Opinion: With so many ‘flavours’ of AI, food and beverage brands must choose wisely
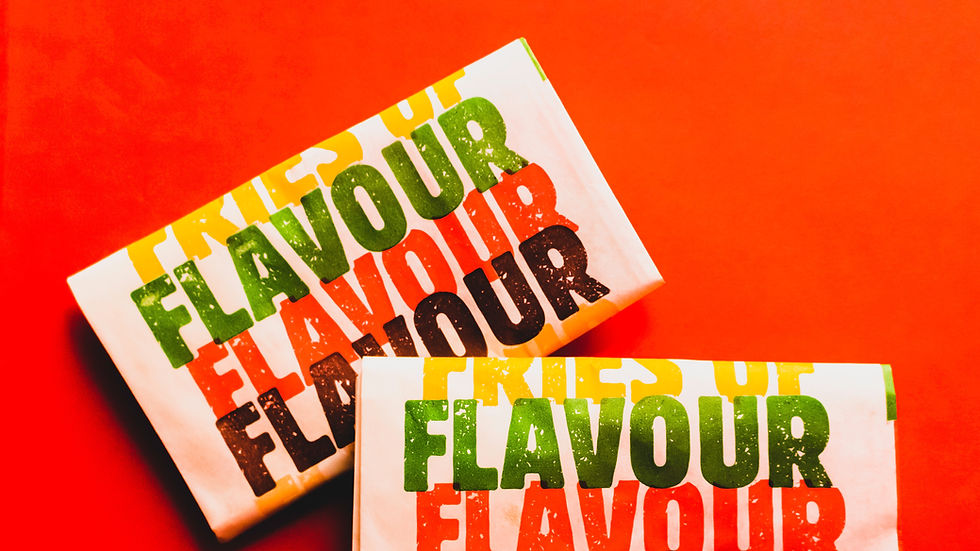

Artificial intelligence (AI) is no longer the stuff of sci-fi – it’s in your warehouse, your supply chain and maybe even your cheese. As AI quietly becomes the secret ingredient behind everything from defect detection to demand forecasting, food and beverage manufacturers must learn to separate the smart from the snake oil. Mohib Rahmani, managing principal and head of the data and AI team (IRIS) at global operations transformation consultancy Argon & Co, spoke to FoodBev about how manufacturers can harness AI, as well as avoid some of the costly pitfalls when incorporating it into their processes.
AI is not a one-size-fits-all solution; it comes in many 'flavours' and food and beverage manufacturers are no exception. In this industry, as in many others, the focus is on narrow AI – designed to excel at specific tasks, like demand forecasting or quality control. For instance, machine learning algorithms can analyse sales patterns to predict demand spikes. Similarly, computer vision systems inspect packaging for defects at high speeds, improving quality assurance.
The real value lies in understanding where AI adds value and where human judgment remains irreplaceable. Take supply chain management, for example. AI can flag a delayed shipment based on real-time data, but a procurement expert is needed to interpret the context. AI needs to empower people, not replace them.
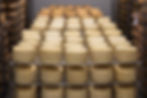
Key factors in selecting AI tools
Choosing the right model can save manufacturers from problems later down the line. The first step is understanding whether your operations need narrow AI or general AI, which is capable of more flexible problem-solving. Most food and beverage operations typically benefit from using narrow AI, for instance, using tools like supervised learning models for predictive maintenance or natural language processing for automating order inquiries.
Before selecting the right AI model, manufacturers should ask themselves: What is the goal? How clean is our data? Is it scalable? Can the model evolve as our operations grow? The answers to these questions will shape which AI model is most suitable for your needs. For instance, if you’re focusing on forecasting, forecasting algorithms work well; but for production, computer vision or anomaly detection tools might be a better fit.
A great example of this is transfer learning. This is where an AI model initially trained on general food production data can be fine-tuned for a niche need – like predicting spoilage for artisanal cheeses. This approach reduces development costs and implementation time.
Predicting changes in consumer demand is where AI comes into its own. Short-term forecasting often uses time-series analysis, a type of machine learning that identifies patterns in historical data. For example, AI can predict a 20% increase in soft drink demand on a hot weekend. By using
demand forecasting with external data, such as weather forecasts, school holidays, social trends and macroeconomic indicators, both manufacturers and retailers can radically improve their short-term and long-term demand planning.
The supply chain areas that benefit most are those where there is an element of human intervention or decision-making where AI can be used to augment humans and remove repetitive tasks, enabling them to focus on the more value-added activities. An alternative example of this would be AI’s role in order processing, where natural language processing can read customer emails and automatically process bulk orders. Paired with soft process automation, this ensures orders are checked, validated and routed faster than with manual methods.
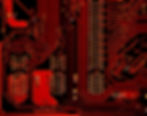
Identifying pitfalls with AI integration
One common pitfall is choosing the wrong type of AI for the job. For instance, using rule-based AI for demand forecasting often falls short because it can’t account for external variables like weather – where machine learning models excel. To avoid this, manufacturers need to match the AI approach to the specific problem they’re addressing.
Manufacturers that adopt AI should not underestimate the importance of communicating with staff about how their AI tools work. Staff must understand how their AI tools work for them to have trust and confidence in the predictions that inform their decision-making. This may also involve the change management aspect of taking the organisation on the journey and highlighting the need for change. For example, in the context of quality control over ingredients, a supervised learning model might predict outputs based on standard wheat properties, but it could fail when there is a slight change in moisture levels. Manufacturers can address this by incorporating unsupervised anomaly detection into their systems to flag deviations in their raw materials for features like moisture content.
Food and beverage manufacturers inexperienced with using AI should start small. A prudent first step might be piloting a simple anomaly detection model for defect detection on a single product line before scaling it up across all lines or using more complicated models.

The future of AI in food and beverage applications
Natural language processing and machine learning are transforming the food and beverage industry by enabling smarter decision-making and automating complex processes. These 'flavours of AI' can tackle the challenge of unstructured data, improve customer engagement and enhance operational efficiency.
There is a real opportunity for success in the case of supplier document intelligence. Here, natural language processing can extract key information from supplier agreements, certifications, and specifications, meaning the entire review process can be automated. For example, natural language processing can identify allergens or sustainability claims hidden in complex documents, meaning compliance obligations can be fulfilled without the need for manual checks. Natural language processing tools can also be used to continuously scan regulatory updates globally and map them to the company’s product portfolio, meaning food and beverage businesses can stay ahead of the curve when it comes to observing their compliance obligations.
Another exciting development is recipe optimisation and ingredient matching. Machine learning models can analyse historical recipe performance, ingredient costs, and nutritional data to suggest optimal formulations. 2025 is shaping up to be a big year for AI in the food and beverage industry, as we see a faster adoption of machine learning models, the promise of reduced waste and healthier, more cost-effective products will soon be realised.
Related news
A meaty debate: The battle over cultivated meat bans
Will cultivated meat find its place on consumers’ plates, or is the industry facing an uphill battle? The Cell base explores.
Opinion: Can regenerative agriculture bridge the gap between cost and sustainability?
As the need for sustainable food systems intensifies, regenerative agriculture emerges as a promising solution. Ethan Soloviev (left),...
Opinion: The basal media bottleneck holding cultivated meat back
Cai Linton of Multus explores how basal media bottlenecks are stalling progress and how innovative solutions can help cultivated meat thrive
Flavour
Opinion: With so many ‘flavours’ of AI, food and beverage brands must choose wisely
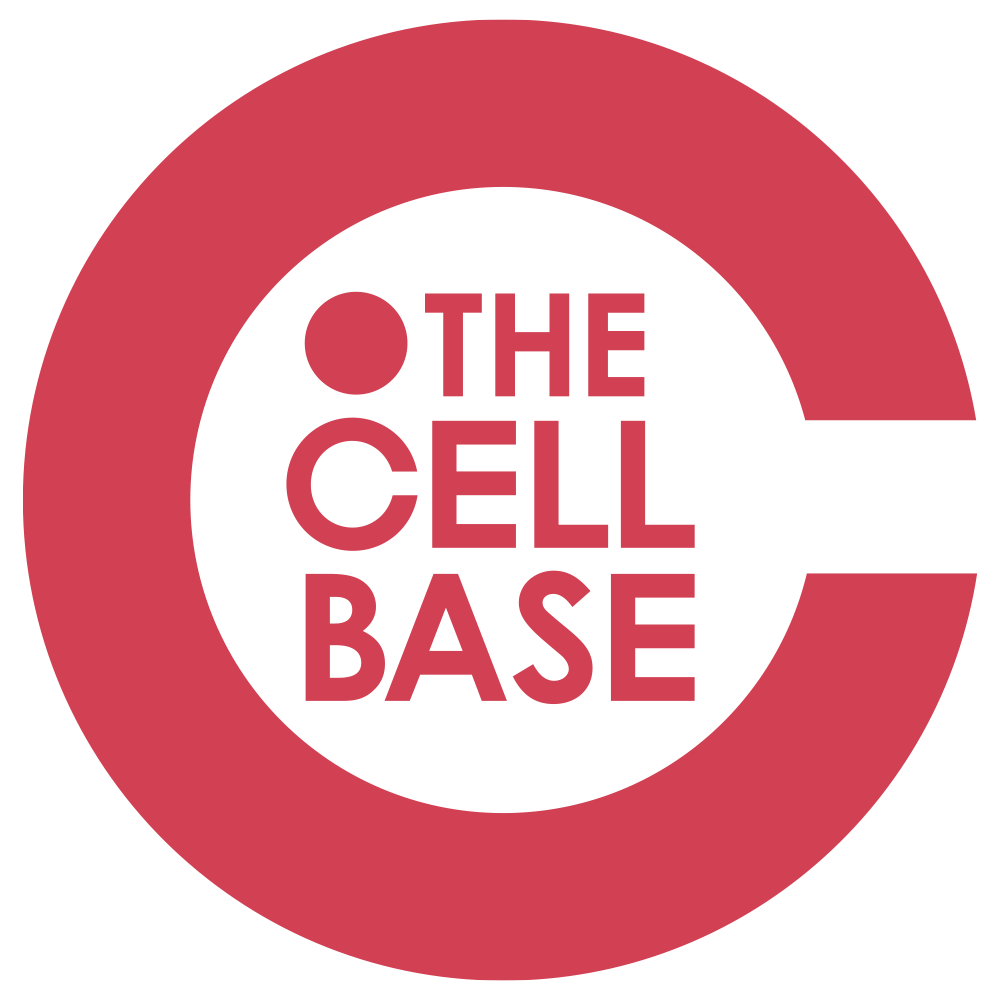
Guest
20 April 2025